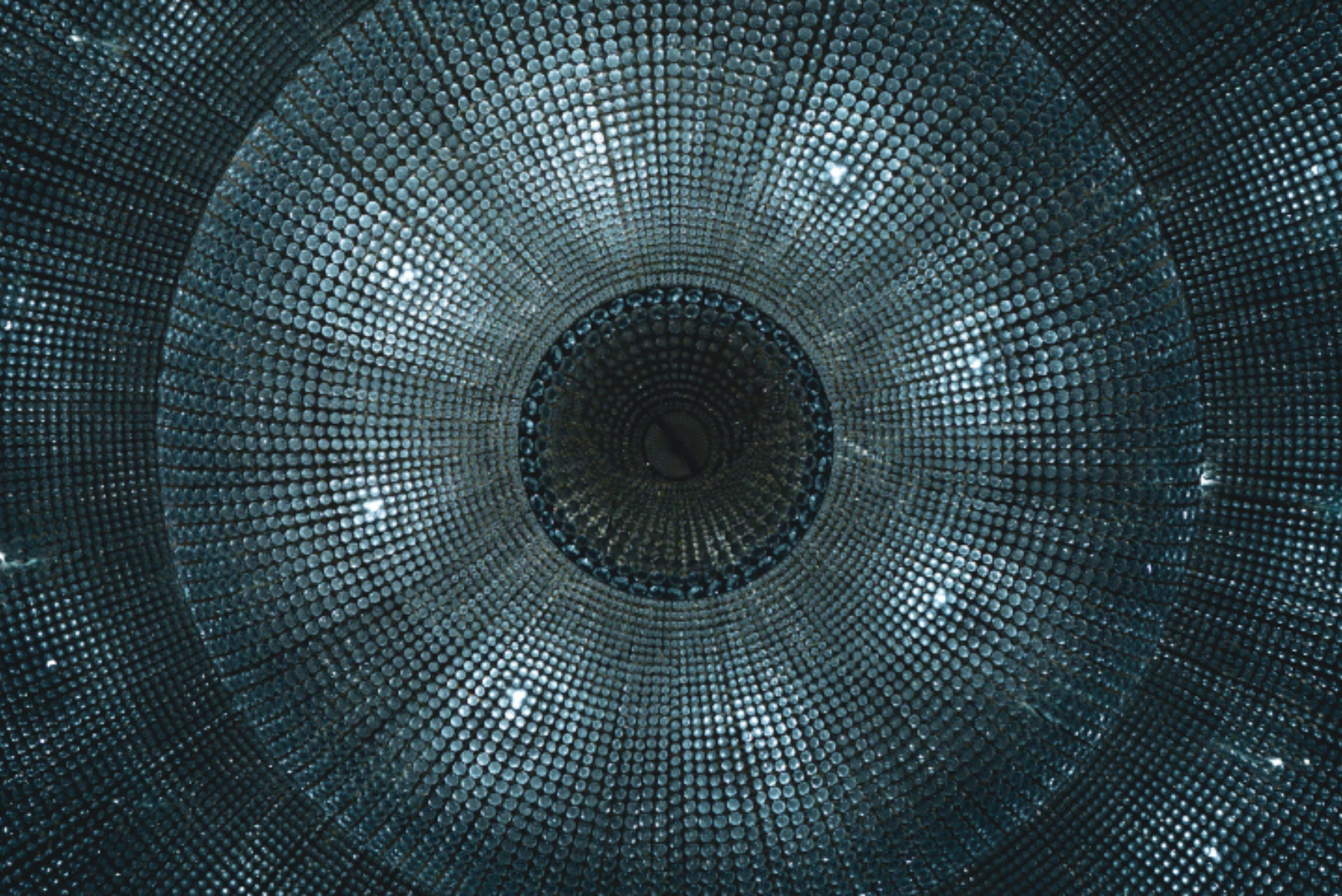
3 keys to achieve the industrialisation of Artificial Intelligence
The adoption of Artificial Intelligence (AI) by companies in all sectors is becoming increasingly widespread. According to the latest report from MarkesandMarkets it is expected that this technology will generate 407 billion dollars by 2027, reaching a compound annual growth rate of 36.2%. Although it is a mature technology, the vast majority of AI models remain in the initial testing phase, without being produced on a large scale, mainly due to the effort required to scale the model.
The lack of progress in the industrialisation of these models means a great inefficiency of resources both for the company and for employees, who do not have access to technologies that could facilitate their daily work. In this context and due to the importance of Artificial Intelligence (AI) in the future of organisations, atSistemas, a 100% Spanish digital services consultancy, highlights three key methods to achieve the industrialisation of AI.
Increasing the data quality, traceability and accessibility
The results obtained by any AI, ML or DL model are directly related to their quality. Therefore, if an organisation intends to industrialise its AI, the first step would be to focus on its databases. These are usually dispersed, and often even in isolated silos, which makes it difficult to use them for large-scale AI models, since if the team only has access to a subset of the data, it will never be able to generate models that reflect the reality of the organisation.
Thus, the objective of the organisation should be to generate data products with higher quality, availability and easier access for the operation, in order to avoid that each area of the company seeks to create its own copy to cover its specific needs and avoid duplication. Once the information has been centralised, it will be essential to guarantee the quality of the data, both from a structural point of view and from a business perspective. In other words, in addition to being correctly filled in, they must be consistent and provide value for employees and the company.
Focusing on multidisciplinary interaction
AI is directly associated with innovation, and the key to innovation is to be able to bring together different perspectives on the same subject, so that they enrich each other and provide a more complete vision. Therefore, the more groups working together, the better the results. This is also essential to achieve the industrialisation of AI, as the global contribution and collaboration of the different teams involved is necessary to achieve an optimal result.
Without this cooperation, it is really difficult to build models that bring added value to the whole organisation and could lead to the problem of generating isolated ecosystems. It is also necessary that the collaboration of the different teams takes place in all cycles, from data ingestion to the implementation of analytics, working in a synchronised way so that the data is available in the shortest possible time, with good quality and easy access.
Creating operating environments
It is not enough just to have good data and the tools to draw useful conclusions for the business. The business must be prepared for the use of that data by creating a robust strategy. The company must identify which of its operations need to be modified to enable the adoption of new technologies, and above all establish standards for the new AI models creation, testing and deployment.
This will make it much easier to replicate and extend new models as they are created. An essential part of the strategy is to establish where the selection of algorithms and the development of models will reside, in the technical or functional teams, and to resource them to achieve greater autonomy without losing control over developments.
Our expert opinion
“Artificial intelligence will have an increasing presence in companies in all sectors as they move forward in their digitisation process. But in order to take full advantage of this technology, companies need to be able to industrialise the process of creating new AI models, reducing the costs of creation and implementing this technology more often in their daily processes,” says Gustavo Eduardo Sandoval, Head of Data, IoT & Smart Industry at atSistemas.